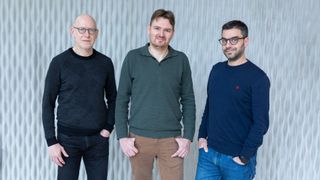
Computational Pathology Group is awarded the 2024 Ammodo Science Award for groundbreaking research
On March 5, 2024, The Computational Pathology Group received the 2024 Ammodo Science Award for groundbreaking research Read more →
The Computational Pathology Group develops, validates and deploys novel medical image analysis methods based on deep learning technology.
On March 5, 2024, The Computational Pathology Group received the 2024 Ammodo Science Award for groundbreaking research Read more →
Daan received recognition for collecting the largest dataset for AI in the diagnosis of basal cell carcinoma to date. Read more →
From May 1st, 2023, Geert Litjens was appointed full professor of AI for analysis of medical images in radiology and pathology at Radboudumc. Read more →