Leander van Eekelen, Enrico Munari, Luca Dulce Meesters, Gabriel Silva de Souza, Muradije Demirel-Andishmand, Daan Zegers, Monika Looijen-Salamon, Shoko Vos, Francesco Ciompi
Abstract
Nuclei detection in histopathology images is an important prerequisite step of downstream research and clinical analyses, such as counting cells and spatial interactions. In this study, we developed an AI-based nuclei detector using the YOLOv5 framework in whole-slide NSCLC cases. Our dataset consisted of 42 PD-L1 stained cases (30 training, 12 test). Four trained (non-expert) readers manually annotated all nuclei (both positive/negative) within regions of interest (ROIs) viewed at 40X magnification. We trained a YOLOv5(s) network on annotations of one reader. Performance was measured using F1 score analysis; hits were defined as being less than 10 um away from annotations.
We evaluate YOLOv5 on the test set by pairing it against all four readers separately. There, YOLOv5 performs excellently, falling within the interrater variability of the four readers: the mean F1 score over algorithm-reader pairs is 0.84 (range 0.76-0.92) while the mean F1 score over pairs of readers is 0.82 (range 0.76-0.86). When we determine the cell count (number of annotations/predictions) per ROI in the test set, agreement of algorithm-reader pairs and reader pairs is equally well aligned: 0.93 (range 0.90-0.97) versus 0.94 (range 0.92-0.96). Visual inspection indicates YOLOv5 performs equally well on PD-L1 positive and negative cells.
In future work, we could extend this detector to additional tissues and immunohistochemistry stainings. Moreover, this detector could be used as a AI-assisted manual point annotation tool: while human readers perform the (context-driven) task of delineating homogeneous regions (e.g. clusters of PD-L1positive stained cells), the detector performs the (local, yet laborious) task of identifying individual nuclei within these regions, providing labelled point annotations.
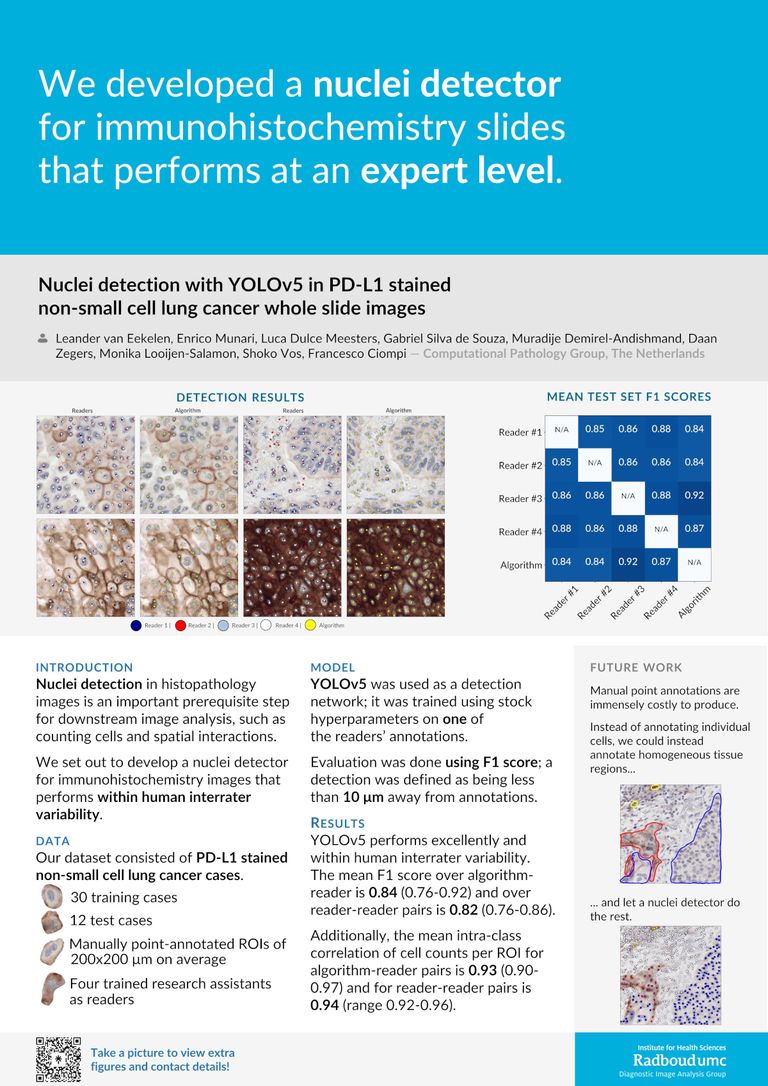