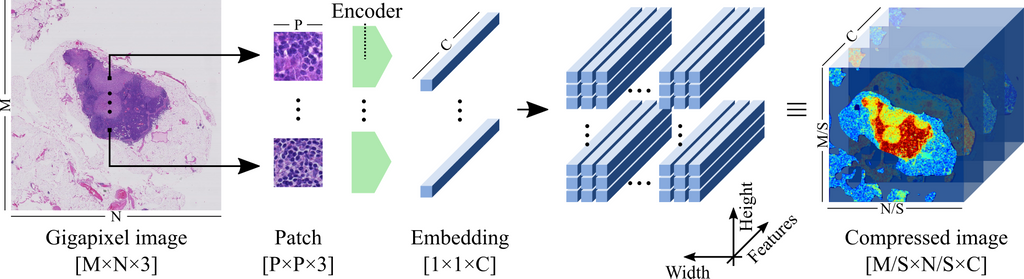
We propose Neural Image Compression (NIC), a two-step method to build convolutional neural networks (CNNs) for gigapixel image analysis solely using weak image-level labels. First, gigapixel images are compressed using a neural network trained in an unsupervised fashion, retaining high-level information while suppressing pixel-level noise. Second, a CNN is trained on these compressed image representations to predict image-level labels, avoiding the need for fine-grained manual annotations. A full description of our method can be found in the journal paper Neural Image Compression for Gigapixel Histopathology Image Analysis and Arxiv link.